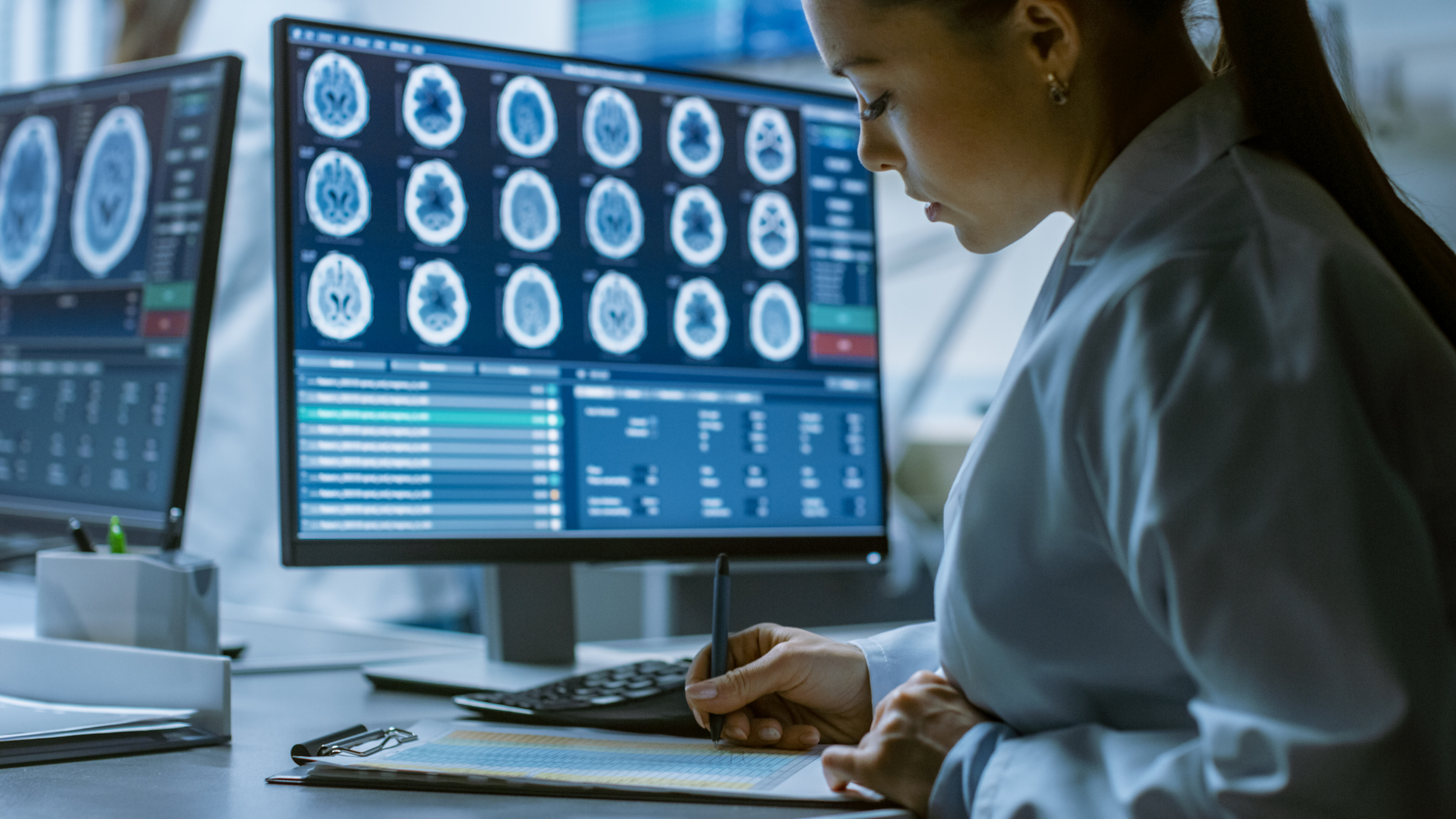
Among the many hottest sectors for synthetic intelligence (AI) adoption is well being care. The Meals and Drug Administration has now accredited greater than 500 AI gadgets—most inside simply the final couple of years—to help docs throughout a spread of duties, from gauging coronary heart failure danger to diagnosing most cancers.
Amid this surge, current research have revealed that AI fashions can predict the demographics of sufferers, together with race, straight from medical pictures, although no distinguishing anatomical or physiological options are evident to human clinicians. These findings have sparked concern that AI programs might discriminate in opposition to sufferers and exacerbate well being care disparity. By the identical token, although, the programs might improve monitoring of affected person outcomes connectable to race, in addition to determine new danger elements for illness.
Calling consideration to those implications, James Zou, an affiliate of the Stanford Institute for Human-Centered AI, and colleagues have penned a brand new “Perspective” article for the journal Science. On this interview, Zou—an assistant professor of biomedical information science and, by courtesy, of pc science and {of electrical} engineering at Stanford—discusses the promise and peril of AI in predicting affected person demographics.
Why is race a doubtlessly problematic idea in well being care settings?
Race is a posh social assemble with no organic foundation. The idea of who is that this or that “race” varies throughout time and completely different contexts and environments; it relies on what nation you are in, what century you are in.
There are different human variables, resembling genetic ancestry and genetic dangers of various illnesses, which are extra nuanced and certain extra related for well being care.
Is AI plausibly discerning organic variations that human clinicians and anatomists have neglected in medical imagery or is it extra seemingly that AI is drawing inferences primarily based on different options in out there information?
Many AI fashions are nonetheless primarily uninterpretable “black containers,” within the sense that customers do probably not know what options and data the AI algorithms are utilizing to reach at explicit predictions. That mentioned, we do not have proof that there are organic variations in these pictures throughout completely different teams that the AI is selecting up on.
We do suppose that the standard and options of the information and of the coaching units used to develop the AI play a serious function. For example, sufferers in a single hospital space or clinic location could also be extra prone to have sure co-morbidities, or different medical circumstances, which even have varied manifestations within the pictures. And the algorithm may decide these manifestations up as artifacts and make spurious correlations to race, as a result of sufferers of a specific racial class occur to stay close to and thus go to that hospital or clinic.
One other chance is systemic technical artifacts, for example from the kinds of machines and the strategies used to gather medical pictures. Even in the identical hospital, there will be two completely different imaging facilities, possibly even utilizing the identical gear, nevertheless it might simply be that workers are skilled in another way in a single imaging room in contrast with the following, resembling on how lengthy to picture a affected person or from what angle. These variances might result in completely different outputs that may present up as systemic patterns within the pictures that the AI correlates with racial or ethnic demographics, “rightly” or “wrongly,” in fact retaining in thoughts that these demographic classes will be crude and arbitrary.
How might AI’s discernment of hidden race variables exacerbate well being care inequalities?
If the algorithm makes use of race or some race proxy to make its diagnostic predictions, and docs will not be conscious that race is getting used, that might result in harmful under- or over-diagnosing of sure circumstances. Trying deeper on the imaging machines and coaching units I discussed earlier than, suppose sufferers of a sure race had been likelier to have scans performed on Kind A X-ray machines as a result of these machines are deployed the place these individuals stay.
Now suppose that constructive instances of, say, lung illnesses within the coaching set for the AI algorithms had been collected largely from Kind B X-ray machines. If the AI learns to think about machine sort when predicting race variables and whether or not sufferers have lung illness, the AI could also be much less prone to predict lung illness for individuals examined on Kind A machines.
In observe, that might imply the individuals getting scanned by Kind A machines could possibly be under-diagnosed for lung illnesses, resulting in well being care disparity.
On the flip facet, how can AI’s skill to deduce racial variables advance objectives of well being care fairness?
AI could possibly be used to watch, assess, and cut back well being care disparity in cases the place medical data or analysis research don’t seize affected person demographic information. With out these information, it’s extremely laborious to know whether or not a sure group of sufferers is definitely getting related care or having related outcomes in contrast with different teams of sufferers. On this manner, ifAI can precisely infer race variables for sufferers, we might use the imputed race variables as a proxy to evaluate well being care efficacy throughout populations and cut back disparities in care. These assessments would additionally feed again in serving to us audit the AI’s efficiency in distinguishing affected person demographics and ensuring the inferences the AI is making will not be themselves perpetuating or introducing well being care disparity.
One other essential profit is that AI can doubtlessly present us with way more granular classifications of demographic teams than the usual, discrete classes of race we regularly encounter. For instance, anybody of Asiatic descent—whether or not from South Asia or East Asia, or whether or not Chinese language or Japanese—is often grouped below one crude umbrella, “Asian,” on customary survey kinds or medical data.
In distinction, AI algorithms usually characterize particular person sufferers on a steady spectrum of variation in ancestry. So there’s attention-grabbing potential there with AI to find out about extra granular affected person subgroups and consider medical companies supplied to them and their outcomes.
Stanford HAI’s mission is to advance AI analysis, training, coverage and observe to enhance the human situation. Study extra.